Topics
What is an artificial intelligence (AI) exchange traded fund?
Artificial Intelligence is simply pattern recognition and an exchange traded fund is a basket of securities that trades on an exchange.
We introduced the world’s first active AI-powered ETF in 2017. That was followed by the launch of an AI Powered International ETF, which brought the same technology to investing outside the US. Our quantitative models are built by EquBot with IBM Watson artificial intelligence.
EquBot’s AI Powered Platform ranks thousands of stocks based on the probability of each company benefiting from current economic conditions, trends and world events, and picks stocks for inclusion in portfolios that have the greatest potential for price appreciation.
To date, the AI Powered Platform is performing much as we had hoped. While you can never assume anything in the stock market – and past performance does not predict future returns, as we all know – the results have been promising.
- AI is pattern recognition.
- ETF is a basket of securities that trades on an exchange.
- AI Powered ETFs are baskets of securities that trade on an exchange and use patterns to pick stocks and other asset classes.
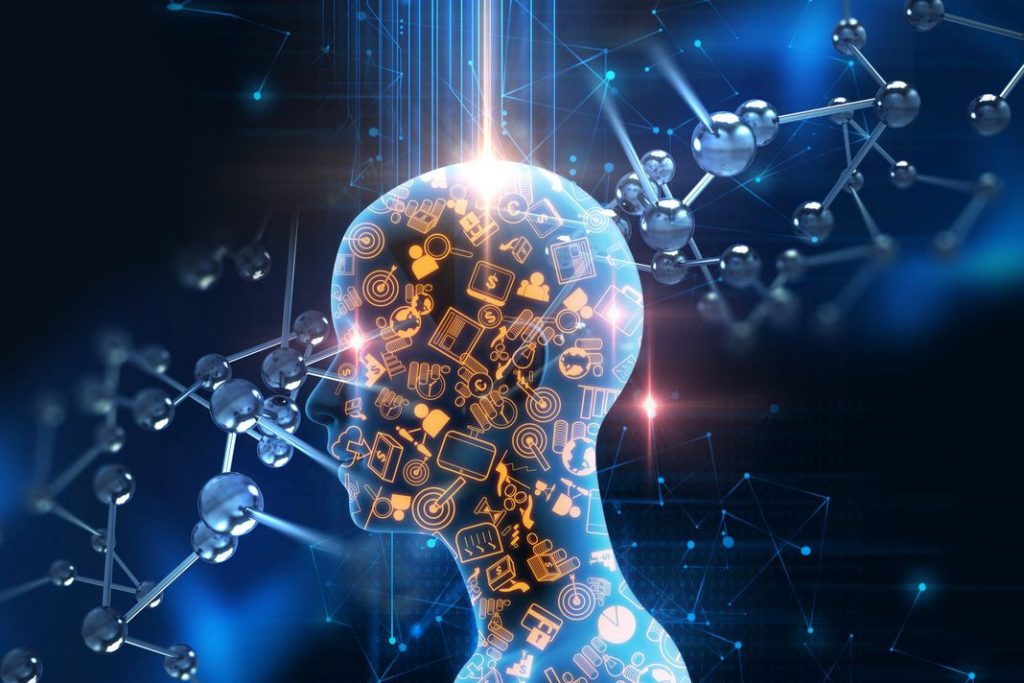
What is the advantage of Artificial Intelligence investing?
- Information Advantage – the ability to process more data including alternative data at a faster pace than what is capable for the human brain.
- Pattern Recognition – discover things that we, as traditional portfolio managers and investors, may not perceive – patterns, relationships, and historical anomalies, for example.
- Removal of human bias – without the implicit biases that can sometimes color the decisions of a human analyst or portfolio management team.
How does an artificial intelligence system pick stocks?
From self-driving cars to cognitive robots, artificial intelligence (AI) is seemingly everywhere these days. It’s increasingly found in the investment world including picking stocks and embedded in hedge fund strategies and Exchange Traded Funds (ETFs) among other products.
As these applications spread, inevitably, a number of misconceptions have sprung up about what AI is, how it works, and what it can and can’t do. A few of the most common ones would include:
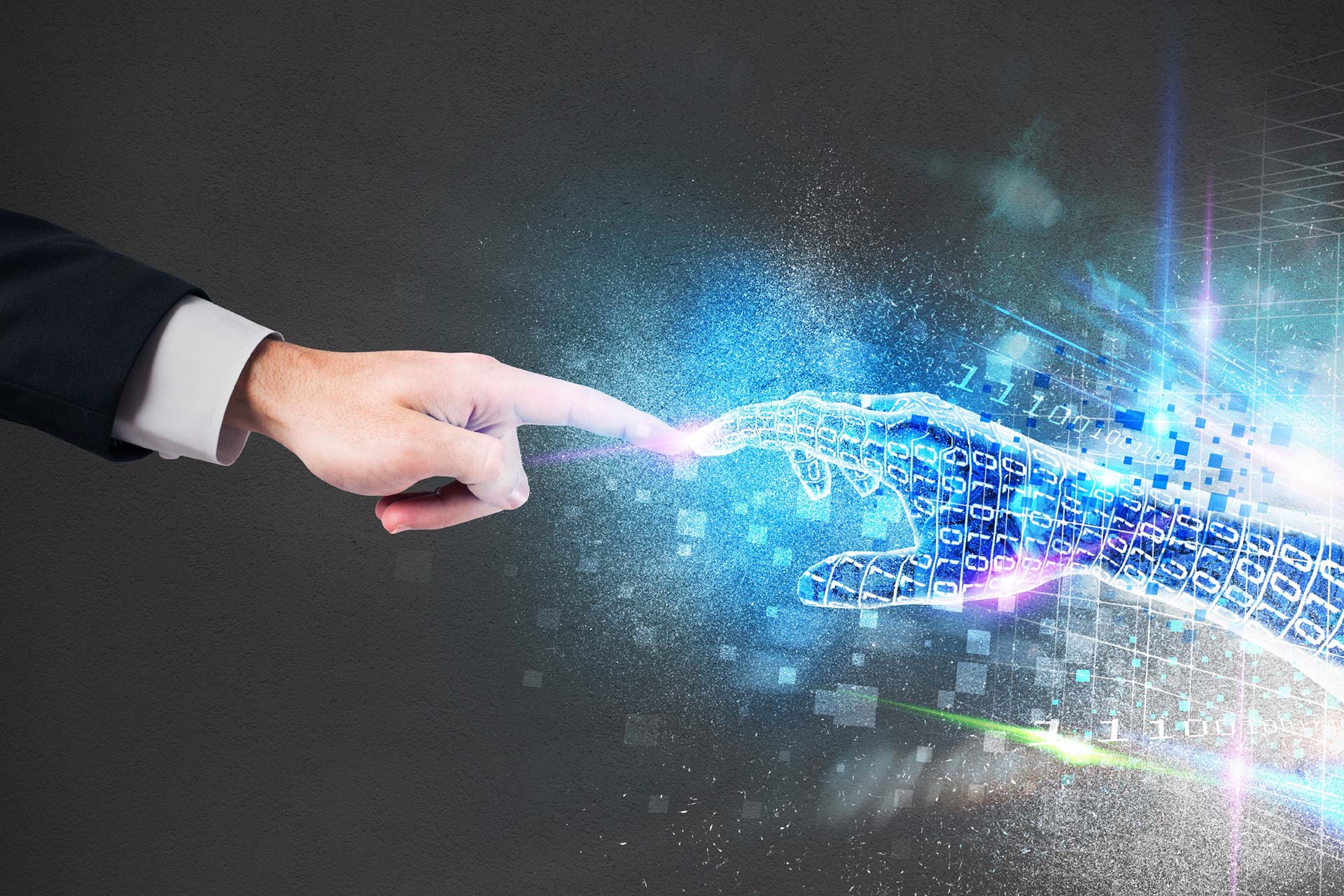
Is it a black box?
Not really. True, the machine learning or AI algorithms that drive the investment process are proprietary and can be complex, but that’s the case with any number of applications that use higher level mathematics to deliver services or to identify customer needs. In our case, we employ a multi-stage process that combines fundamental and qualitative analysis to pick stocks and other assets. This includes using the IBM Watson computing platform to sort through millions of millions of articles and massive amounts of unstructured alternative data to build predictive models on over 15,000 globally traded companies.
Effectively, we’re employing artificial intelligence to augment or replace the portfolio manager or stock picker to process more market data and generate more objective investment decisions given a set of investment criteria.
How does artificial intelligence reach a decision?
This is a frequent criticism of AI – it might deliver the desired outcome but no one knows how it got there. This is particularly critical in picking stocks, where performance attribution is an important metric. But explainable AI systems can be built that allow for sufficient transparency to understand how the algorithms pick a stock or asset. Further, the data sets that are acted on by AI are determined by humans; those, in turn, broadly reflect pre-determined investment goals. The algorithm then advances by uncovering patterns in a kind of trial and error, a reinforced learning process that is directed towards a specific end – picking stocks to outperform a market index, for example. It may discover things that might elude a traditional portfolio manager – patterns, relationships, and historical anomalies, for example. Once identified, these patterns can be observed by humans as well, adding transparency.
Most importantly, with an AI powered ETF the decisions made by the algorithm are there to be seen every day in the publicly-reported holdings.
Is the explainable AI system in charge then?
The goal of any explainable AI powered investment system is to combine the best qualities of technology with human experience and insight. The algorithms themselves are designed by individuals to operate within certain parameters and to achieve well-defined goals – they are essentially recognizing patterns and predicting outcomes. Adding machine intelligence allows them to learn along the way, and to improve, but it doesn’t alter the fundamental purpose. Algorithms created to enhance the investment process or designed to pick stocks won’t suddenly wander off and start playing chess or Go, for example. They’ll try to improve the process for which they were designed.
Eliminating bias in the investment process is an important consideration, but it is not the same as eliminating all human input, or forgoing the opportunity for a human portfolio manager to analyze and oversee the results. Like computers themselves, the algorithms make it possible to do more, faster – well beyond the capability of any individual portfolio manager or team of analysts – both they are subject to human oversight.
- AI Stock Picking and AI Powered ETFs do not use a black box method, but instead use explainable and transparent AI.
- Explainable AI and AI Powered ETFs use methods such as reinforcement learning which is a trial and error method.
- The AI Powered System is not in charge, it is investment and technology professionals who train the system and set the objectives.
- Using a data driven approach for AI sock picking and AI Powered ETFs removes the human bias from the investment process.
What is AI and what does it have to do with investing?
Like many innovative technologies, artificial intelligence (AI) has moved through multiple stages of usability, public understanding and media hype. It’s going to save the world… it’s going to destroy the world… it’s going to make chess-playing humans obsolete… it’s going to provoke a deeper understanding of the game… it’s coming for my job… it’s going to make my work much easier. Any and all of these have been conventional wisdom, a pattern that holds true for a multitude of innovations.
We work in AI, and we have seen something similar in our corner of the world, which focuses on markets and investing. For some, it may seem that AI operates at a point where mathematics and mysticism intersect, in fact what we’re doing is conceptually quite simple: we are creating explainable AI algorithms – step-by-step procedures for solving a problem or accomplishing some end – with the objective of identifying investment opportunities. When an algorithm is then combined with a powerful computing AI platform, such as an IBM Watson, you can include many millions of “steps” in the investment process. But, in the end, you still have to have some idea of what you mean by an investment “opportunity.”
You can add a further wrinkle to this through what’s known as “machine learning” – a process that uses statistical techniques to help an algorithm improve performance without the introduction of additional programming from an external source. In effect, the “machine” learns by doing, though it is still moving towards an optimal outcome as defined by a person.
This is where things can get a bit more mysterious. There was an old saying in the tech business that “software is its own proof.” In other words, it was not always possible to understand how every aspect of a complex program would interact with every other aspect – and how they collectively would interface with a complex and constantly changing real world environment. What we could ultimately observe was whether it worked or not, which brings up another key challenge for AI: for this technology, it’s not just arriving at the solution that’s vital; it’s critical to understand how that solution was reached.
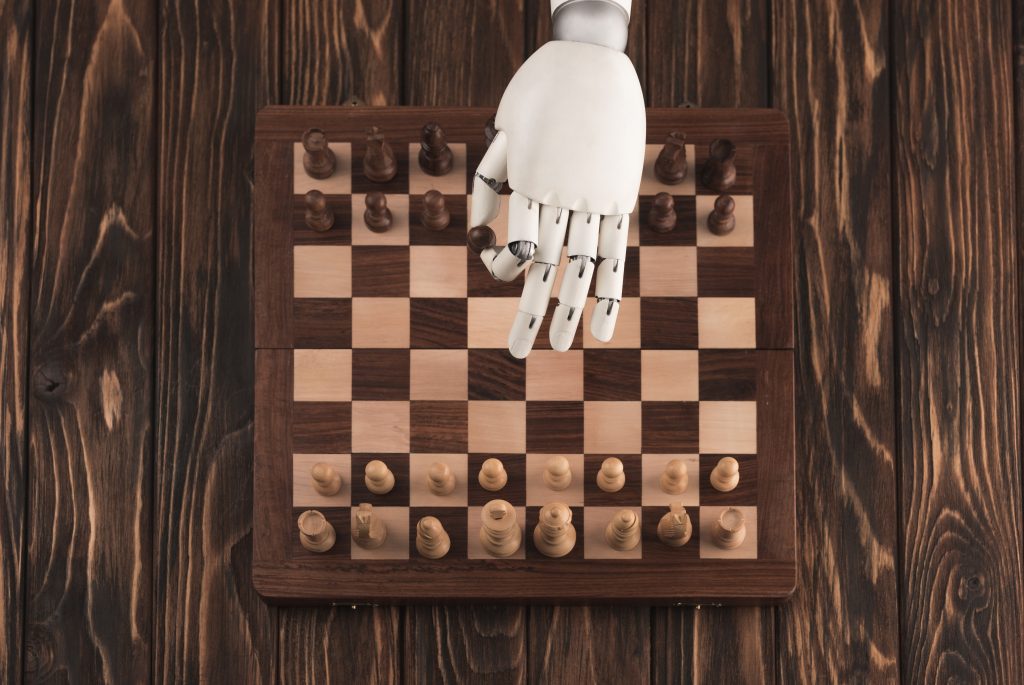
When a computer first beat a human in chess, it did so mainly on the strength of computational ability – it could analyze more possibilities faster and identify an optimal outcome. But it played the game within the known parameters. A few years later when a computer beat a human Go player, it did so in part by uncovering entirely new patterns and possibilities. In investing, we want the explainable AI algorithms to understand what makes a stock, a market, or an asset class attractive. We want them to incorporate a mathematical understanding of the objectives of the investing process (and the AI fund, and, ultimately, the investor). But we also want it to discover things that we, as traditional portfolio managers and investors, may not perceive – patterns, relationships, and historical anomalies, for example.
In practice, this requires a process that incorporates both fundamental and quantitative analysis. It means developing the AI technology to manage “unstructured alternative data” – the massive amounts of information incorporated in the news, or conveyed through social media. There are more than 15,000 globally traded companies that are potential investments. With a market of that scope, realistically, pouring over and intelligently analyzing this alternative data on an ongoing basis is something only AI machines can do. Making sense of it all, discarding the noise and identifying opportunities, is the role of AI, which does all of this without the implicit biases that can sometimes color the decisions of a human analyst or portfolio management team. Improving the process as it goes along requires the application of sophisticated machine learning techniques.
When the algorithms are used to build a transparent vehicle like an AI powered Exchange-Traded Fund (ETF), the decisions they make are publicly available every day for everyone to see, in much the same way an active human manager can highlight their buy and sell decisions or in the way an index provider can publish its respective rulebook. Thus, the “mystery” of AI is really no mystery at all; rather it is a process that can be explained, watched and understood, but one that has the capability to sift through orders of magnitude more data in order to identify opportunities to invest in mispriced equities around the world.
It was the science fiction writer Arthur C. Clarke who said that, “Any sufficiently advanced technology is indistinguishable from magic.” AI may be somewhat new to the investment world, but it’s not magic. It’s an investment process not that different from what has gone before. And the goal is one shared with millions of investors over the years: to outperform a given benchmark.
- AI algorithms – step-by-step procedures for solving a problem or accomplishing some end – with the objective of identifying investment opportunities.
- Machine Learning – a process that uses statistical techniques to help an algorithm improve performance without the introduction of additional programming from an external source.
- AI can not only analyze more possibilities faster and identify an optimal outcome, but also uncover entirely new patterns and possibilities.
- AI investing can discover things that we, as traditional portfolio managers and investors, may not perceive – patterns, relationships, and historical anomalies, for example.
- AI investment technology can manage “unstructured alternative data” – the massive amounts of information incorporated in the news, or conveyed through social media.
- AI investing can sift through orders of magnitude more data in order to identify opportunities to invest in mispriced equities around the world.
- AI-powered investing tools can be a powerful differentiator, time saver, and means of uncovering new and previously undiscovered opportunities to better serve clients and deliver meaningful value.
How Will AI Impact the Future of Investing?
It is difficult to understate how disruptive artificial intelligence (AI) will be, and in many ways already has been, to the investment industry. Put simply, AI’s ability to parse billions of pieces of data including new alternative data, allows for the formulation of unbiased investment insights in ways the human mind cannot.
When we look to the next evolution of asset management and the role that AI will play, we consider three key factors that will continue to drive the use of AI and ML (machine learning) in the investment process:
Why is data a key factor?
First, data. 90% of all data in existence was created in the past two years, and that pace will only be increasing going forward. Consequently, decision-making and data analysis will become more of a challenge for the traditional fundamental researcher or manager. Having the ability to quickly assimilate and understand all of this data and make unbiased decisions will therefore not only be advantageous to the forward-thinking asset manager, it will be essential. And, despite the concerns that “human jobs will be lost to the machines,” we believe it’s unlikely that AI will operate as a complete replacement for human managers and analysts. What it may mean is tougher times for those firms that do not consider the ways in which AI may help them improve their investment processes, and competitive advantages for those that use AI to more efficiently manage increasingly overwhelming amounts of market data.
Why is innovation important?
Second, innovation around AI-powered products and approaches continues to develop. The introduction and availability of AI-powered investment products like ETFs and tools continues to expand, opening new doors for investors. Further, as the category continues to grow, opportunities for asset management firms to develop ever more customized AI-driven investing approaches will be a major boon for firms as they seek to anticipate their clients’ needs.
Why are technologies critical?
Third, to the point above, financial institutions are seeing these technological enhancements being applied and reported across other industries, and their interest is only rising. Asset management has historically been somewhat behind the curve when it comes to incorporating new and emerging technologies, but we’re seeing more direct investment by financial services firms into AI and ML with the goal of making better investment decisions and becoming more efficient.
Clearly, the adoption and implementation of AI in the investment industry is going to be essential for firms looking to stay on the cutting edge.
- Data is being created at an exponential pace which is a tailwind for AI investing.
- Innovation such as AI Powered ETFs will create new investor tools.
- Direct investments by financial institutions in AI and ML is increasing.
How AI is Disrupting the Investment Industry?
The following text is from a response from the EquBot leadership team to the media on AI Powered Investing and ETFs
Artificial intelligence (AI) continues to build its presence across global industries so its progression into the asset management sector is only a matter of time. Before we explore the development of investment industry disruption let’s level set on what AI actually is. For this piece we should think of AI as a field in computer science that builds intelligence into electronic systems. These AI systems are able to perceive data environments, learn, and take actions to achieve its objectives. This definition presents a compelling value proposition for AI versus traditional portfolio management systems in light of today’s growing data environment. The key technological advantage of AI investment platforms is their overall flexibility to appropriately process perpetually shifting market data and identify opportunities.
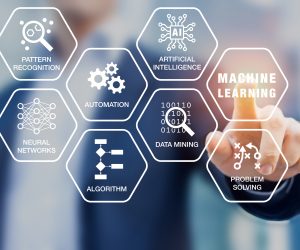
AI can help us better understand what to trade, and how to trade it. Important AI capabilities include: consuming, learning, and aggregating growing volumes of traditional and alternative data across mediums, continually adjusting portfolio risk based on observed market signals (removal of rigid factor-based criteria), and the ability to connect new market signals to derive an optimized portfolio free of human bias.
Investors must understand that not all AI is created equal. There is a myth that AI investment picks cannot be fully observed let alone explained. The truth is that AI investment platform design will vary significantly, and in turn influence performance. High performing systems should appropriately action structured and unstructured data in a timely manner as well as appropriately manage erroneous or blatantly fake financial news.
Many early stories of AI investment systems described the brute force design of jamming massive data sets into the conceptual “black boxes” and allowing the machine to produce a series of recommendations. Better solutions exist and are more suitable for the explosion of available investment data and the demand for system operational observability. The systems unable to deconstruct results may be prone to introducing unintended investment risks into a portfolio rather unintelligently. The more transparent and higher performing AI data processing systems will drive increased flows into the space. We anticipate an influx of AI investment products so investors should look to understand how traditional and alternative data is processed and what the AI investment process entails as they shift out of their (soon to be) legacy investment vehicles.
Even with the recent AI technological developments and improved commercial accessibility, many investors will struggle to understand the potential value in well-designed AI investment solutions (limited AI track records available). Early adopters of this emerging technology stand to be the largest beneficiaries. Platform users will benefit from growth in their investment knowledge base facilitated by machine learning algorithms. Investors will likely experience tailwinds from incremental investments as AI success stories continue to emerge. About 90% of electronic data was created in the past two years, and in two years from now we will likely be saying the same thing: AI will become a necessary tool for global investors.
AI is already disrupting the ETF industry through AI powered ETFs and it is having spillover effects into other investment vehicles. EquBot AI Powered ETFs are running on AI platforms using IBM Watson that work around the clock to process traditional and alternative data on over 15,000 publicly traded global companies. Millions of market signals, news articles, and social media posts are processed to produce thousands of hypothetical test portfolios which are further distilled down into daily trade recommendations. We observe an increase in the number of usually secretive and tight-lipped hedge fund managers admitting to using AI after these AI ETFs launched. Others will eventually catch on that more cost-efficient and effective asset management structures exist with the use of AI investing.
We do not feel AI investing will immediately replace human managers and analysts but will allow them to more efficiently manage the overwhelming amounts of market data. As AI investment products challenge broad benchmark market indices we anticipate the same type of shift we saw from taxis to ride share programs like Uber and Lyft. AI brings humility and potential economic upside to investors willing to admit they aren’t able to keep up with the growing snowball of information. It is very much an admission that even the most sophisticated investors don’t know what combinations of datasets are best suited to build target investment portfolios. We propose a start with AI investment solutions.
- The key technological advantage of AI investment platforms is their overall flexibility to appropriately process perpetually shifting market data and identify opportunities.
- AI investing can help us better understand what to trade, and how to trade it.
- AI investment platform users will benefit from growth in their investment knowledge base facilitated by machine learning algorithms.
- AI will become a necessary tool for global investors as the sheer amount of data explodes at an exponential rate.
- AI investing is already disrupting the ETF industry through AI powered ETFs and it is having spillover effects into other investment vehicles.
- Millions of market signals, news articles, and social media posts are processed to produce thousands of hypothetical test portfolios which are further distilled down into daily trade recommendations.
- AI investment products will challenge broad benchmark market indices.
What are the Roadblocks of AI Backtesting?
The following text is from a response from the EquBot leadership team to the media on AI backtesting
Institutional investors must satisfy various manager selection criteria before funding a new strategy. Historical investment track record is very important in these processes, but backtesting is often substituted to accommodate newer quantitative strategies. Complex investment management systems offer various forms of factor criteria to reconstruct portfolio constituents and analyze relative performance for specified periods of time to satisfy backtesting requirements. Since we have a perfect vision of what has transpired in the markets, many technical investment products suffer from the “overfitting” syndrome associated with backtesting. Talented quantitative investment professionals can aggregate multiple signals and can generate amazing backtest returns for strategies going back decades. Sophisticated investors need to question if this is the right approach for a perpetually changing investment environment relative to more complex investment solutions that leverage real time data.
Investment research has indicated the majority of data has been created in the past two years. Given the growth of data we believe that this statement will hold true two years in the future. A vast majority of the data growth will come from alternative data sources. Alternative data sources like announcements distributed across business wires, social media, and videos can have different degrees of impact on financial markets. AI investment strategies analyzing sentiment, intent, magnitude, and credibility from digital media seem to offer a compelling value proposition in being able to analyze the volumes of data and derive conclusions in a timelier manner when compared to traditional investment managers. Many instinctually feel the importance of these newer alternative data sources, but how can they be validated?
Web data is archived, so why not overlay the same backtesting methods on these unstructured alternative data sets relative to the holdings in the aforementioned tests?
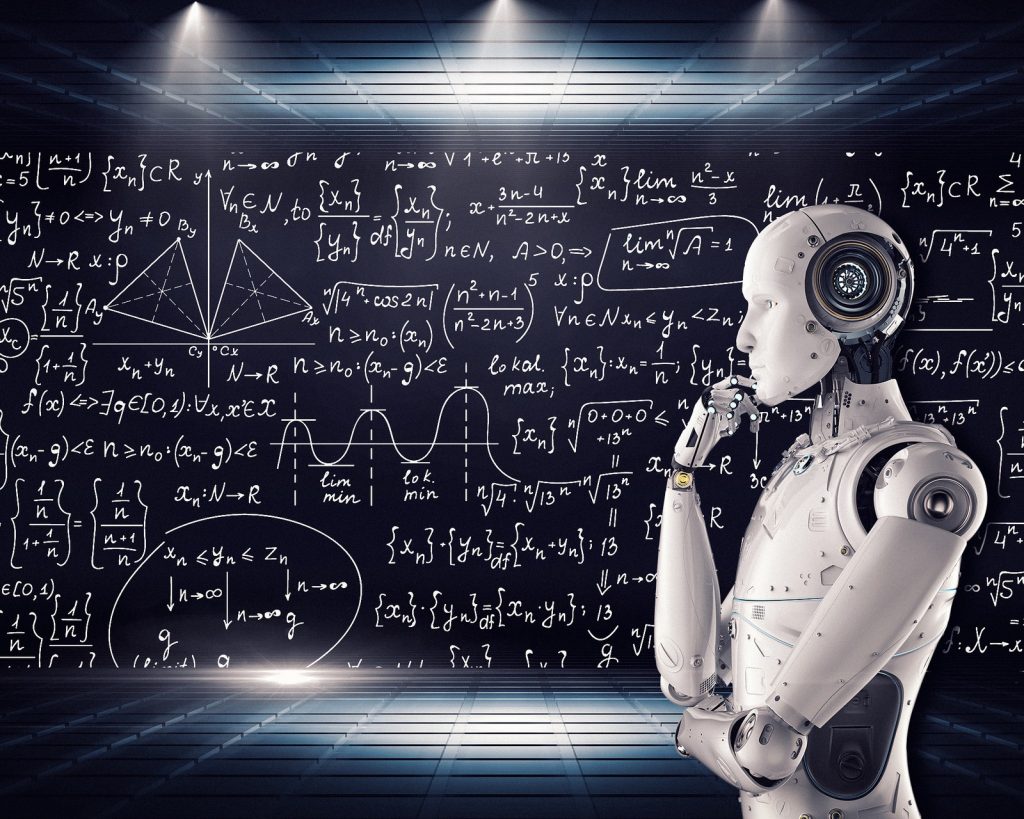
As a result of the explosion of data, archived information loses detail.
Time stamps will become muddled if you go back a handful of years. When seconds can move the market and impact the profitability of a trade, applying unstructured data signals to backtest results can be misleading. The most reasonable expectation of backtesting with respect to newer AI investment strategies should involve matching historical data characteristics to the frequency of trading. As such, if you see a daily active rebalancing AI strategy, a 30 year backtest should be heavily scrutinized. If you are using daily signals, then a reasonable backtest should involve datasets so far as the daily detail can be derived.
The alternative data explosion is being driven by new sources of information. Similarly, we will see many historical data sources disappear. This is a critical requirement for any investor looking to allocate money into the AI investment space. Maintaining an understanding on how an investment manager is handling the explosion of new data, and monitoring the ongoing credibility of existing information is important. A flexible solution should be in place, and should be openly discussed to confirm if the technological advantage of the manager will hold.
The jury is still out to determine the optimal measure of the potential efficacy of a highly quantitative AI investment strategy. Similar to the procedure of understanding an investment manager process, investors should include a data management process criteria to their quantitative AI investment manager searches. We understand firsthand how track records and backtests can cause friction in the current institutional investment environment but remain optimistic. EquBot is an advocate of backtesting investment strategies for periods with commensurate duration of observed signals and trading activity, and data management discussions. No one can say for sure what the investment information environment will look like 30 years in the future, but we can say with confidence that portfolios 30 years ago were not facing the impact of social media posts and other alternative data like they are today.
- The information is perpetually shifting
- Data continues to grow at an exponential rate
- As a result of the explosion of data, archived information loses detail
- Newer AI investment strategies should involve matching historical data characteristics to the frequency of trading
- Monitoring the ongoing credibility of existing information is important
- AI investment strategies analyzing sentiment, intent, magnitude, and credibility from digital media seem to offer a compelling value proposition in being able to analyze the volumes of data and derive conclusions in a timelier manner when compared to traditional investment managers
- Investors should include a data management process criteria to their quantitative AI investment manager searches