Like many innovative technologies, artificial intelligence (AI) has moved through multiple stages of usability, public understanding and media hype. It’s going to save the world… it’s going to destroy the world… it’s going to make chess-playing humans obsolete… it’s going to provoke a deeper understanding of the game… it’s coming for my job… it’s going to make my work much easier. Any and all of these have been conventional wisdom, a pattern that holds true for a multitude of innovations.
My colleagues and I work in AI, and we have seen something similar in our corner of the world, which focuses on markets and investing. For some, it may seem that AI operates at a point where mathematics and mysticism intersect, in fact what we’re doing is conceptually quite simple: we are creating algorithms – step-by-step procedures for solving a problem or accomplishing some end – with the objective of identifying investment opportunities. When an algorithm is then combined with a powerful computing platform, such as an IBM Watson, you can include many millions of “steps” in the process. But, in the end, you still have to have some idea of what you mean by an investment “opportunity.”
You can add a further wrinkle to this through what’s known as “machine learning” – a process that uses statistical techniques to help an algorithm improve performance without the introduction of additional programming from an external source. In effect, the “machine” learns by doing, though it is still moving towards an optimal outcome as defined by a person.
This is where things can get a bit more mysterious. There was an old saying in the tech business that “software is its own proof.” In other words, it was not always possible to understand how every aspect of a complex program would interact with every other aspect – and how they collectively would interface with a complex and constantly changing real world environment. What could ultimately observe was whether it worked or not, which brings up another key challenge for AI: for this technology, it’s not just arriving at the solution that’s vital; it’s critical to understand how that solution was reached.
When a computer first beat a human in chess, it did so mainly on the strength of computational ability – it could analyze more possibilities faster and identify an optimal outcome. But it played the game within the known parameters. A few years later when a computer beat a human Go player, it did so in part by uncovering entirely new patterns and possibilities. In investing, we want the algorithms to understand what makes a stock, a market, or an asset class attractive. We want them to incorporate a mathematical understanding of the objectives of the investing process (and the fund, and, ultimately, the investor). But we also want it to discover things that we, as traditional portfolio managers and investors, may not perceive – patterns, relationships, and historical anomalies, for example.
In practice, this requires a process that incorporates both fundamental and quantitative analysis. It means developing the technology to manage “unstructured alternative data” – the massive amounts of information incorporated in the news, or conveyed through social media. There are more than 15,000 globally traded companies that are potential investments. With a market of that scope, realistically, pouring over and intelligently analyzing this data on an ongoing basis is something only machines can do. Making sense of it all, discarding the noise and identifying opportunities, is the role AI, which does all of this without the implicit biases that can sometimes color the decisions of a human analyst or portfolio management team. Improving the process as it goes along requires the application of sophisticated machine learning techniques.
When the algorithms are used to build a transparent vehicle like an Exchange-Traded Fund (ETF), the decisions they make are publicly available every day for everyone to see, in much the same way an active human manager can highlight their buy and sell decisions or in the way an index provider can publish its respective rulebook. Thus, the “mystery” of AI is really no mystery at all; rather it is a process that can be explained, watched and understood, but one that has the capability to sift through orders of magnitude more data in order to identify opportunities to invest in mispriced equities around the world.
Will AI ever completely replace human beings in the investment management process? No, nor will it replace human beings in the financial advisor space. People will always need people to help guide them in reaching their financial goals, but for both investment managers and advisors, the application of AI-powered investing tools can be a powerful differentiator, time saver, and means of uncovering new and previously undiscovered opportunities to better serve clients and deliver meaningful value.
It was the science fiction writer Arthur C. Clarke who said that, “Any sufficiently advanced technology is indistinguishable from magic.” AI may be somewhat new to the investment world, but it’s not magic. It’s an investment process not that different from what has gone before. And the goal is one shared with millions of investors over the years: to outperform a given benchmark.
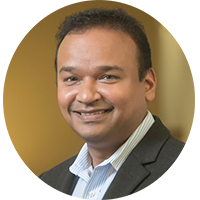
Chida Khatua
co-founder and CEO of EquBot